Will Science be able to Predict Everything in the Future?
- Author: Cristobal S. Cruz Psychology B.S. Editors:
- Dec 25, 2016
- 8 min read
Predicting Complex Systems
Imagine you are in possession of a complex device (such as a highly sophisticated smartphone) that can know everything about the inner-workings of a system. For example, this device could measure your town’s weather with 100% accuracy. From measuring the precise humidity to predicting the exact arrival of a storm, the device can measure every input that could possibly influence the weather of the town. Any information regarding the weather we could possibly want is computable from a collection of algorithms stored inside the device that are situation specific—covering all possible configurations of inputs (i.e. the causal and instrumental variables in question) to the weather.
Obviously, no such device exists with our current science and technology. Yet in principle it appears, assuming science and technology continue to progress as has been historically the case, that mankind will eventually arrive at such technology—or at least should. However, there seems to be a precipice we encounter when trying to construct this technology that may be indicative of its insolvability. This problem appears because of the way inputs are integrated into the system in question. Before moving too fast by this idea, let us linger on it for a moment while considering the system of the town’s weather, or, the town’s weather system.
The Contribution of Inputs
For the weather-measuring device we have in mind, the inputs cannot contribute to their outputs in the same manner as say, inputs to a clock can. There are a fixed set of inputs that are available for a clock’s initial conditions and moreover these fixed inputs are constant. They do not vary in frequency or intensity as they are integrated into the clock system. For example, the minute hand on the clock is programmed to move a certain length every minute. That program will never change—it has been instructed to repeat one process (the frequency) that has only one type of impact (the intensity) on the system. That program might stop someday, but this is inconsequential because the natural factors that contribute to its failure (e.g. battery life) operate outside the clock system (not relevant to the clocks proper functioning) so they need not be considered. Nonetheless, because the input of the program is static, we can reliably create devices that predict what the clock will do. In a sense, a digital clock would be a mechanical clock’s prediction device! The main point is that you can achieve a basic understanding of the clock’s mechanics and proceed to make 100% accurate predictions about its future behavior.
The same point cannot be made for the town’s weather. The inputs integrate into the town’s weather system in such a fashion that they (and their corresponding outputs) are affected by earlier integration of inputs. This is because the frequency and intensity of inputs into the town’s weather system at one point in time can be affected by prior frequencies and intensities of inputs. For example, how much (frequency) and how forcefully (intensity) it rains depends on factors (such as humidity or wind speed) that are themselves dependent on how much and how forcefully the rain is coming down—even if they are dependent in the most subtle of ways. The device used to measure these inputs cannot be conceptualized in the same way the digital clock can. The digital clock has constants as inputs—the inputs remain static in terms of their frequency and intensity. Our device that measures the town’s weather, however, only knows constants as a minority of the inputs it receives.
Sensitive to Initial Conditions
Because these inputs are in constant flux, we cannot predict with any confidence the precise configuration of the weather in the next instant. What would indeed be necessary for that kind of prediction is the detailed knowledge of the earlier inputs. To summarize in a somewhat oxymoronic fashion: to create a device with a perfectly exhaustive list of inputs and outputs for the town’s weather, we would need information about how their “constants change” (i.e. how the inputs that otherwise would be constant vary over time). This information could, in principle, be known if we had information about the initial conditions of the system. The phrase sensitive to initial conditions reflects this observation. With knowledge of a system’s initial conditions we could trace every possible configuration of inputs from the beginning of the system to the current moment and then beyond that! This way, we would be aware of how the “constants change” at every moment. This also reveals to us that only when inputs are changing in frequency and intensity will there result a system that is sensitive to its own initial conditions.
Nonetheless, by following a system’s flow of inputs that are highly sensitive to initial conditions, we would have the power to ensure accurate prediction. As you might suspect, gaining precise knowledge of a system’s initial conditions or the system’s entire history of inputs and outputs is quite the arduous task—especially considering the weather has existed longer than our species has been around to study it! So how are we to understand and work with systems that operate with such inevitable unpredictability?
Tough Predictions with Sensitivity to Initial Conditions
These initial conditions relentlessly stab at the power predicting in real time—making attempts often appear foolish and sometimes banishing disciplines as soft sciences or even pseudosciences. Although sciences vary in the degree to which they can be confident in their predictions, this is not to say we should dismiss any predictions that are not held with 100% confidence. Take meteorologists for example. Even though their predictions are often wrong, they still are able to keep their profession; this leads one to believe there must be something amuck going on behind the scenes. Yet, we still happily rely on weather predictions when planning events, directing plane routes, and other weather dependent activities. This is an example of a case where we are just approaching the power of our imagined perfect weather measuring device and still reap benefits.
The weather forecasters inch closer to 100% accurate predictions, but inevitably fall short due to lack of information—even (and as we shall see, sometimes especially) if that information is causally “subtle” in nature. Perhaps they never will achieve 100% accuracy—in fact, it does not appear likely that they will. It happens be the case, ironically, that in order to know everything about the future, we must know everything about the past. And it does not seem plausible that we will be creating a time traveling device anytime soon. But we haven’t squeezed out all the optimism from our sponge of prediction yet. To get around not having precise knowledge about past conditions, we can still make inferences about the past in attempts to re-create it. Indeed, this is what we do when predicting the weather globally—a significantly more formidable system in terms of complexity than a single town’s weather system.
How Initial Conditions Block Information
Despite the pessimism surrounding accurate prediction, it is important to keep in mind that we can still access the utility of prediction by approaching 100% accuracy. Unknown initial conditions block access to certain information, but still leave us with a lot to work with! This might lead one to think that the amount of information known about a system should be proportional to our predictive power regarding that system; however, this is not the case! Even very small gaps in knowledge regarding prior or initial conditions may result in huge differences in output. For instance, imagine that we are in control of the various constantly spinning input dials that control the various mechanisms we collectively refer to as weather. Let us imagine that each of the dials range from 1-100. We could spin each dial and allow them to go on spinning forever and let the resulting patterns of weather unfold. But we must keep in mind that we would arrive at very different patterns of weather if we started spinning one of our dials from a slightly different position—say, starting at “3” instead of “4.” The seemingly minuscule difference between 3 and 4 doesn’t intuitively warrant suspicion that the resulting patterns will be radically different, but perhaps it should. Because the current weather patterns are so reliably dependent on the initial conditions of our dials, if knowledge of the dial’s initial conditions were withheld we could only infer so much about how the resulting weather patterns will continue to change.
The effect of initial conditions can also be demonstrated every time you roll a pair of die. Even if you attempt to drop the dice in the same way, it is very unlikely that rolling them a second time will result in their landing in the same configuration (except perhaps if you dropped them mere centimeters from the floor). Try it. Drop two dice about a foot from the ground and look at the resulting configuration of dice. Now try to get them to land in the same configuration. You will notice that this is an almost impossible task regardless of how still you attempt to keep your hand or how carefully you take into consideration the original position of the dice in your hand as you let them fall to the ground. You will soon come to realize that the slightest deviation from the way you initially dropped the dice results in vastly different configurations of dice on the ground. In the same way the final configuration of dice is intimately connected to the precise initial conditions of your hand, the prediction of the weather is connected to its own initial conditions. This effect is often referred to as the butterfly effect which is based on the idea that the effects of a butterfly flapping its wings (the initial or prior conditions) in Japan might down the road incite a hurricane in North America (the complex output).
Can We Ever Hope to Make 100% Accurate Predictions?
Consider all the intricacies involved in predicting behavior of complex (indeed chaotic as some researchers refer to them) systems. It hasn’t become any clearer how such a useful prediction device could be created. We now know that this device somehow has to overcome the problem of backtracking every step of prior inputs to arrive at the starting points—a type of inferential backtracking, if you will, that gets us to the initial conditions. Not all hope is lost because the resources we have approach such a device’s power (such as the weather forecasters who use computers to run statistical models for weather prediction). Despite the progress, there are some boundaries we cannot cross. To say that we can build such a perfect prediction device is to essentially say that we can build a time machine. And the impossibility of building a time machine seems a much easier pill to swallow than the impossibility of prediction.
Rethinking the Origins and Future of Complexity
Entertain the idea that we are thinking about creating this device in the wrong way. Perhaps we need to let such a device come about naturally—maybe it already has come about naturally. Now, we might not expect a device that can perform inferential calculations to occur naturally. Certainly, there exist seemingly insurmountable difficulties that Mother Nature would have to overcome to create such a device. However, Mother Nature was (and is) unconcerned with the difficulties surrounding prediction and so nonetheless attempted to construct such a device of her own.
Mother Nature decided to use the facilitating mechanism of natural selection to construct her device. This mechanism allowed for the evolution of squishy machines made of water and proteins that can extract information from the present environment and couple it with information about the past in real time to make predictions about the future. This evolutionary process has slowed, for now, and left us with the human brain—a highly effective inferential machine that has a readily accessible storage of information about the past, ways to configure that information with the present, and ways to manifest the result of this combined information as some output.
This leads directly into the next blog topic regarding cognitive/psychological science and how the phenomenon these sciences study is relatable to the underlying neurophysiological mechanisms. This is important to consider; because when psychologists do their research, they must distinguish between theories that are and are not compatible with the neuroscience. Scientists must tread carefully in order to not fall victim to generating theories around findings that are superfluous to or are false positives of true cognitive mechanisms. Constructing theories that have strong neural counter-parts can be one of the best ways to get around this problem. Conceptualizing theories like this might suggest that the lower or more fundamental science has some power over the softer or less descriptive science. This leaves open the question of whether we should expect that focus on neuroscience and ultimately a complete our understanding of it would do away with softer sciences such as psychology. Despite the inseparable union between neuroscience and psychology there still exists a chasm that keeps the two sciences distinct. As we shall see, sensitive dependence on initial conditions, as well as information processing has much to do with this chasm.
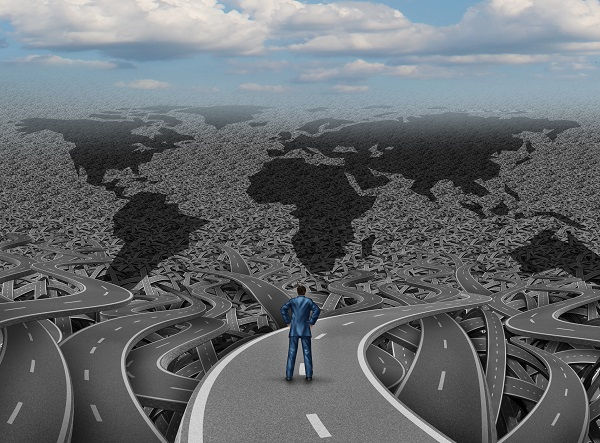
Comments